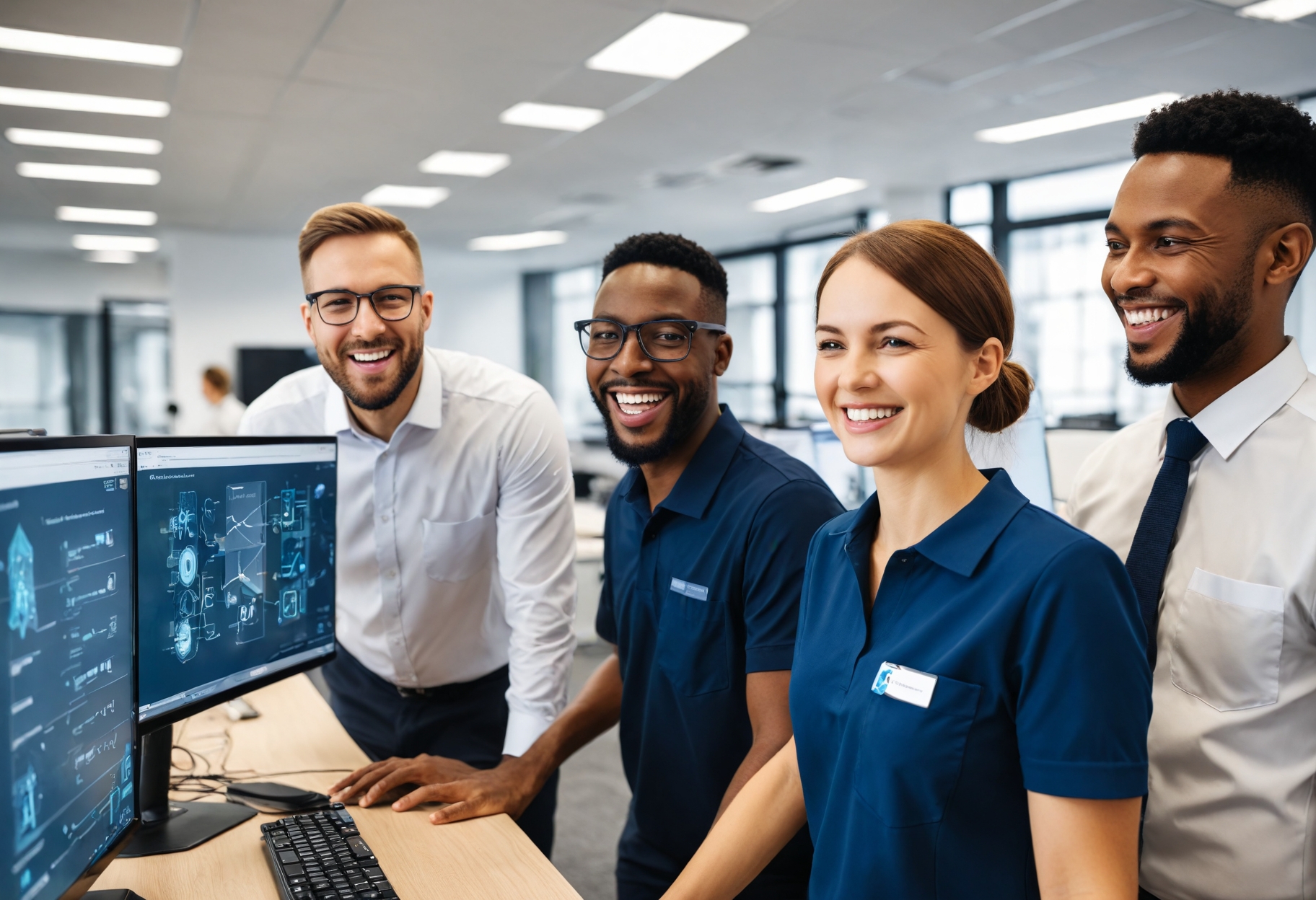
The Shift from Reactive to Predictive Maintenance
Traditional maintenance methods—like reactive and preventive maintenance—often fall short in optimizing asset performance and reducing downtime. Predictive maintenance, enabled by lifecycle management tools, takes a data-driven approach, predicting issues before they lead to costly breakdowns. By using real-time data and advanced analytics, predictive maintenance empowers businesses to manage assets efficiently, extend equipment lifespan, and achieve significant cost savings.
How Predictive Maintenance Works with Lifecycle Management Tools
- Data Collection through IoT Sensors Predictive maintenance relies on IoT sensors embedded in assets to collect real-time data on performance indicators like temperature, vibration, and energy consumption. This continuous data flow allows maintenance teams to monitor equipment health at all times, identifying potential issues before they escalate.
- Analysis of Performance Data for Early Issue Detection With lifecycle management tools, data is analyzed to detect performance patterns that may indicate wear, misalignment, or other issues. Advanced analytics can pinpoint minor deviations, helping teams address them before they lead to more severe problems, minimizing unplanned downtime.
- Automated Alerts and Maintenance Scheduling Lifecycle management systems generate automated alerts based on performance thresholds, notifying teams when assets require maintenance. These systems optimize scheduling, ensuring that maintenance is done at the most convenient time, avoiding disruptions during peak operations.
- Prolonged Asset Life and Reduced Replacement Costs Predictive maintenance helps prolong asset life by preventing overuse and ensuring timely repairs. Reduced wear and tear result in fewer breakdowns, lowering the need for replacements and conserving capital expenditure.
- Resource Optimization and Improved Productivity By scheduling maintenance only when needed, predictive maintenance reduces unnecessary servicing, optimizing resource allocation. Teams can focus on critical assets, while automated alerts ensure that essential equipment remains operational, boosting productivity.
Benefits of Predictive Maintenance with Lifecycle Management
- Reduced Downtime and Increased Uptime Predictive maintenance minimizes unplanned downtime by allowing teams to address issues before they impact operations. Companies using predictive maintenance report a 20-30% increase in equipment uptime, resulting in greater productivity and more reliable service.
- Lower Maintenance Costs By performing maintenance only when it’s necessary, companies can reduce costs associated with unnecessary repairs and excessive parts replacements. Predictive maintenance saves up to 30% in maintenance costs by enabling more strategic, data-backed maintenance schedules.
- Improved Safety and Compliance Lifecycle management tools ensure that assets remain in safe operating conditions, reducing the risk of accidents. Predictive maintenance allows companies to meet compliance requirements more efficiently, as equipment is consistently maintained within regulatory standards.
- Enhanced Asset Utilization Predictive maintenance helps maximize asset utilization by ensuring assets are maintained only when needed, rather than following arbitrary schedules. This approach prevents over-servicing, allowing for optimized asset deployment across operations.
- Long-Term Savings through Extended Asset Lifespan With predictive maintenance, assets remain in peak operating condition for longer, reducing wear and tear and delaying replacement costs. Businesses can gain more value from their investments by extending asset life and reducing capital expenditure.
Stages of Predictive Maintenance in the Product Lifecycle
- Monitoring and Data Collection In this initial stage, IoT sensors and lifecycle management tools gather data on asset performance, including operational patterns and environmental conditions. Continuous monitoring provides a baseline to detect anomalies early.
- Data Analysis and Issue Detection Lifecycle management systems analyze historical and real-time data, using machine learning algorithms to detect early signs of potential issues. Teams can then proactively schedule maintenance based on actual asset health rather than fixed intervals.
- Maintenance Scheduling and Execution Once potential issues are detected, lifecycle management tools generate automated alerts, enabling maintenance teams to plan repairs at optimal times. By scheduling maintenance in advance, companies avoid the costs associated with emergency repairs.
- Review and Optimization After each maintenance event, data is reviewed to refine predictive models, improving accuracy and effectiveness over time. This continuous improvement loop ensures that maintenance practices evolve alongside asset performance data, further enhancing reliability.
Use Case Examples
- Manufacturing: Reducing Equipment Downtime A manufacturing company implemented predictive maintenance for its heavy machinery using lifecycle management tools with IoT sensors. By analyzing vibration and temperature data, the system alerted maintenance teams of impending issues. The company reduced equipment downtime by 40% and saved significant costs in emergency repairs, boosting overall productivity.
- Healthcare: Ensuring Reliability of Medical Equipment A healthcare provider deployed predictive maintenance for critical medical devices, such as MRI machines and ventilators, using lifecycle management tools. IoT sensors monitored equipment condition, alerting the team to maintenance needs before equipment failure. This proactive approach reduced unplanned equipment downtime by 25%, ensuring reliable availability of essential devices for patient care.
- Logistics: Optimizing Fleet Maintenance for Cost Savings A logistics company integrated predictive maintenance for its fleet of delivery vehicles. Sensors monitored engine performance, tire pressure, and mileage, enabling predictive scheduling of maintenance before breakdowns occurred. The company experienced a 30% reduction in maintenance costs and improved fleet availability, resulting in higher on-time delivery rates.
Analytics: Measuring the Impact of Predictive Maintenance
- Reduction in Unplanned Downtime Companies implementing predictive maintenance experience up to a 50% reduction in unplanned downtime, as issues are detected and addressed before they escalate.
- Increase in Asset Life Expectancy Predictive maintenance extends asset life by 20-30%, allowing companies to delay costly replacements and reduce capital expenditure over time.
- Lower Maintenance Frequency and Costs With fewer emergency repairs and optimized maintenance scheduling, companies report a 25-30% reduction in maintenance costs, alongside improved productivity and resource allocation.
Conclusion: The Future of Maintenance with Predictive Lifecycle Tools
Predictive maintenance, empowered by lifecycle management tools, is transforming how companies maintain and manage assets. By using real-time data and advanced analytics, predictive maintenance minimizes downtime, lowers costs, and extends asset life. For companies aiming to achieve operational efficiency and maximize asset value, adopting predictive maintenance is an essential investment in a sustainable, data-driven future.