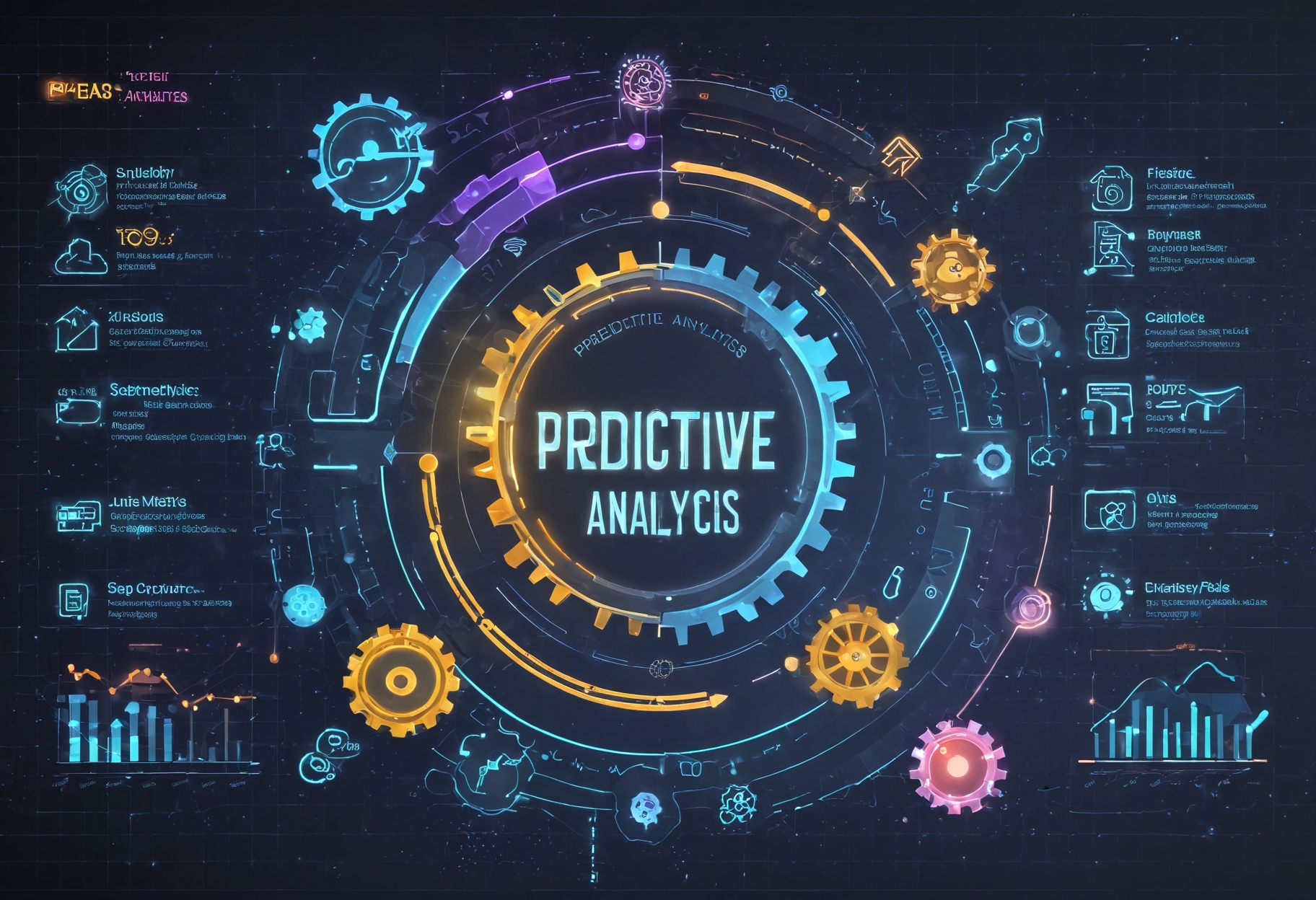
The Value of Predictive Analytics in Product Lifecycle Management
Predictive analytics has become a powerful tool in product lifecycle management, offering insights that help companies anticipate challenges and optimize processes. By analyzing historical data and identifying patterns, predictive analytics enables organizations to make informed decisions at every lifecycle stage. From forecasting demand to preventing equipment failures, predictive analytics supports a proactive approach that enhances lifecycle efficiency, reduces costs, and improves overall product quality.
How Predictive Analytics Optimizes the Product Lifecycle
- Demand Forecasting for Accurate Inventory Management Predictive analytics helps companies forecast customer demand by analyzing historical sales data, seasonal trends, and market shifts. This foresight enables precise inventory planning, reducing the risk of stockouts and overstocking, and aligning production with real customer needs.
- Optimized Production Scheduling and Resource Allocation Predictive models can optimize production schedules by forecasting when and where resources are needed most. This level of insight allows companies to allocate equipment, personnel, and materials efficiently, minimizing idle time, avoiding production bottlenecks, and maximizing productivity.
- Preventive and Predictive Maintenance for Asset Longevity Predictive analytics identifies patterns in equipment performance, allowing companies to schedule maintenance based on actual asset conditions rather than fixed intervals. This proactive approach minimizes unexpected downtime, extends asset life, and optimizes maintenance costs by reducing unplanned repairs.
- Enhanced Quality Control Through Defect Prediction By analyzing data from previous production runs, predictive analytics can identify risk factors for defects. These insights allow companies to address potential quality issues early, adjusting processes or materials to maintain high standards and reduce the likelihood of costly recalls.
- Improved End-of-Life Planning and Sustainability Predictive analytics can also support end-of-life planning by forecasting when a product is likely to become obsolete or experience reduced demand. This data allows companies to plan responsible disposal, recycling, or repurposing strategies that minimize waste and align with sustainability goals.
Use Case Examples
- Consumer Electronics: Reducing Stockouts with Demand Forecasting A consumer electronics company used predictive analytics to forecast demand for its popular devices based on historical sales and market trends. This approach reduced stockouts by 30% and improved inventory turnover, ensuring products were available when and where customers needed them.
- Manufacturing: Minimizing Equipment Downtime with Predictive Maintenance A manufacturing company implemented predictive analytics to monitor machinery performance and predict maintenance needs. By scheduling maintenance only when necessary, the company reduced downtime by 25%, extended equipment lifespan, and lowered maintenance costs.
- Automotive: Enhancing Quality Control by Predicting Defects An automotive manufacturer used predictive analytics to identify factors contributing to production defects. By adjusting production processes based on these insights, the company reduced defect rates by 20%, improving product quality and customer satisfaction.
Analytics: Measuring the Impact of Predictive Analytics on Product Lifecycle Optimization
- Reduction in Inventory Holding Costs With accurate demand forecasts, companies experience a 20-30% reduction in inventory holding costs, as predictive analytics allows for leaner stock levels and reduced excess inventory.
- Decrease in Unplanned Downtime and Maintenance Costs Predictive maintenance reduces unplanned downtime by up to 25-35%, as companies can address equipment issues proactively, extending asset life and optimizing maintenance resources.
- Improvement in Product Quality and Customer Satisfaction By preventing defects and ensuring consistent quality, predictive analytics contributes to a 15-20% improvement in customer satisfaction, as products meet or exceed customer expectations.
Conclusion: Predictive Analytics as a Strategic Advantage in Lifecycle Management
Predictive analytics empowers companies to make proactive, data-driven decisions that enhance product lifecycle efficiency and quality. By forecasting demand, optimizing maintenance, and preventing defects, predictive insights allow organizations to anticipate and address lifecycle challenges effectively. For companies committed to operational excellence, implementing predictive analytics in product lifecycle management offers a strategic advantage that supports sustainable growth, improved product quality, and higher customer satisfaction.