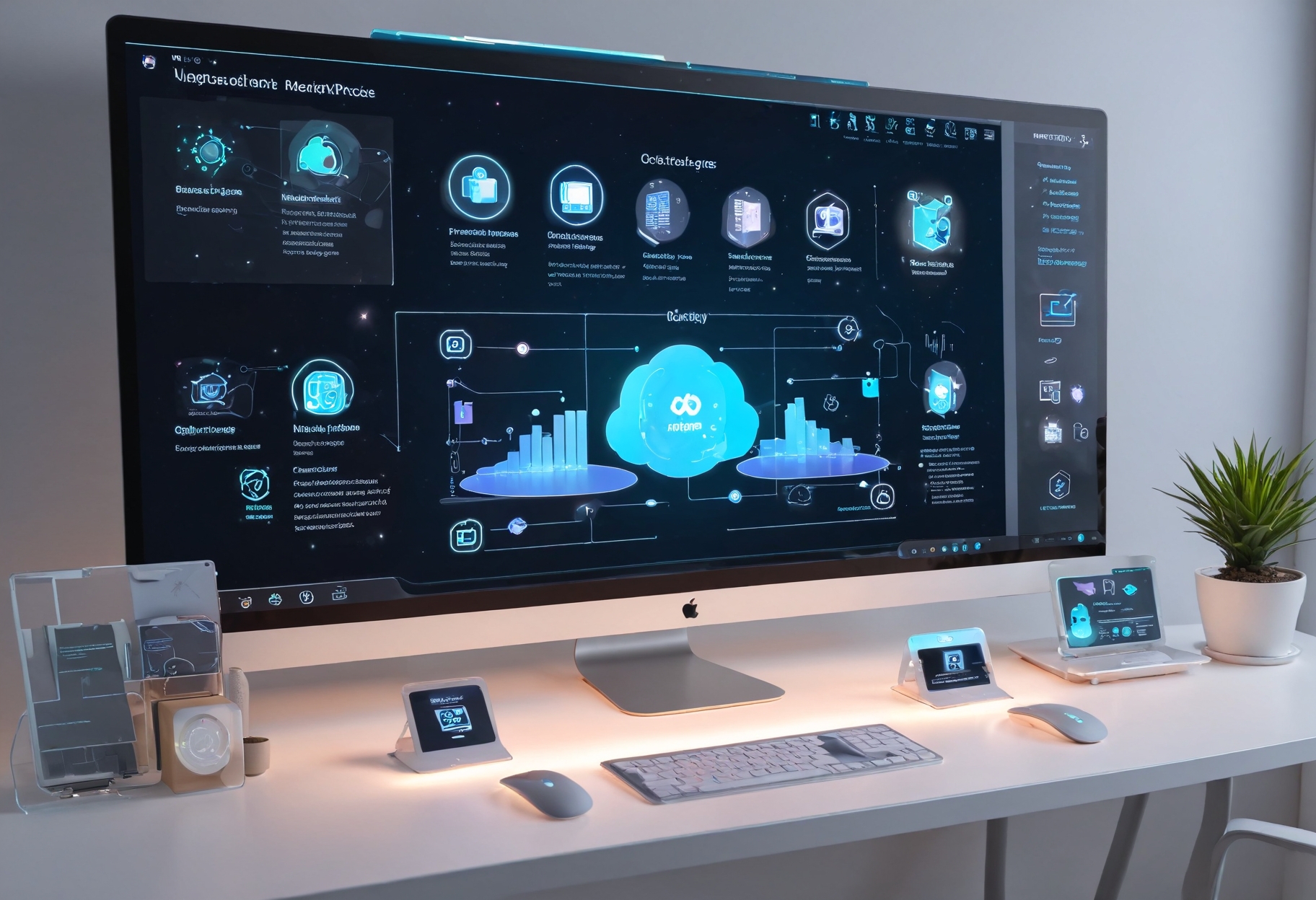
The Impact of Machine Learning on Product Lifecycle Management
Machine learning brings advanced predictive capabilities and process automation to product lifecycle management. By analyzing large volumes of data and identifying hidden patterns, ML enables companies to optimize workflows, reduce costs, and improve decision-making. From predictive maintenance to demand forecasting, machine learning offers powerful tools for improving efficiency across all stages of the product lifecycle, allowing organizations to stay agile in a competitive market.
How Machine Learning Enhances Lifecycle Efficiency
- Predictive Maintenance for Reduced Downtime Machine learning algorithms analyze historical data from equipment, identifying patterns and predicting potential failures. This proactive maintenance approach allows companies to schedule repairs before issues arise, reducing downtime, extending asset life, and optimizing maintenance resources.
- Automated Quality Control and Defect Detection ML-powered image recognition and data analysis tools help detect defects in products during manufacturing. By automating quality control, ML reduces human error, ensures consistency, and improves product quality, supporting a higher standard of output across production cycles.
- Demand Forecasting and Inventory Optimization Machine learning algorithms analyze sales data, customer behavior, and market trends to forecast demand accurately. This predictive capability allows companies to align inventory with demand, minimizing stockouts, reducing excess inventory, and improving overall inventory efficiency.
- Enhanced Product Design and Customer Insights By analyzing customer feedback and usage patterns, ML algorithms provide insights that guide product design improvements. ML enables companies to develop products that better meet customer needs and preferences, shortening design cycles and enhancing market relevance.
- Process Optimization Through Anomaly Detection Machine learning models can identify anomalies in production processes, helping teams detect inefficiencies or errors in real time. By flagging irregularities, ML enables quick adjustments, reducing waste and ensuring that production runs smoothly, which supports lean and efficient operations.
Use Case Examples
- Automotive: Reducing Maintenance Costs with Predictive ML Models An automotive company used machine learning to analyze engine performance data and predict when components would need maintenance. This predictive maintenance approach reduced unexpected breakdowns by 30% and optimized repair schedules, resulting in significant cost savings.
- Consumer Electronics: Enhancing Quality Control with ML-Powered Defect Detection A consumer electronics manufacturer implemented machine learning for automated quality checks on its assembly line. ML-based image recognition detected defects early, allowing for timely corrections and reducing defect rates by 25%, leading to better customer satisfaction and fewer returns.
- Retail: Aligning Inventory with Demand Through ML Forecasting A retail company used machine learning to forecast product demand based on historical sales and seasonal trends. By aligning inventory with predicted demand, the company reduced excess stock by 20% and minimized stockouts, improving profitability and optimizing storage costs.
Analytics: Measuring the Impact of Machine Learning on Lifecycle Efficiency
- Reduction in Downtime and Maintenance Costs Companies using ML for predictive maintenance report a 25-35% reduction in downtime and maintenance costs, as maintenance is scheduled based on actual asset condition rather than set intervals.
- Improvement in Quality Control Accuracy With ML-driven quality checks, companies experience a 20-30% improvement in quality control accuracy, as automated systems reduce errors and maintain consistent standards.
- Decrease in Excess Inventory and Holding Costs Machine learning-driven demand forecasting enables a 15-25% reduction in excess inventory, as forecasts are aligned more closely with real-time demand, minimizing holding costs and waste.
Conclusion: Machine Learning as a Driver of Lifecycle Efficiency
Machine learning offers a strategic advantage in lifecycle management by enabling predictive insights, automating processes, and optimizing resources. From predictive maintenance to demand forecasting, ML empowers companies to improve efficiency, reduce costs, and respond proactively to lifecycle demands. For organizations focused on innovation and operational excellence, implementing machine learning in product lifecycle management is essential for achieving smarter, data-driven outcomes.