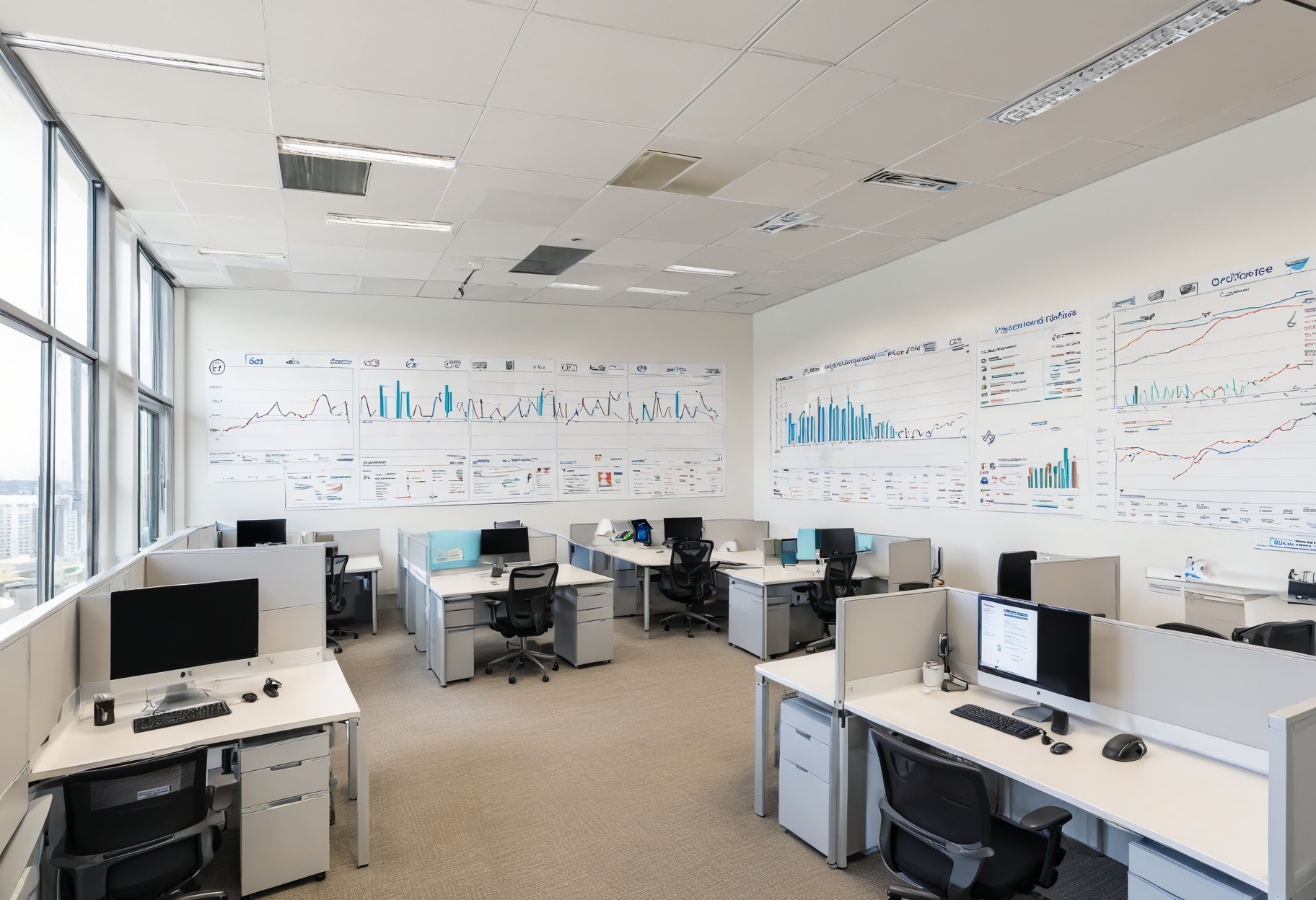
The Role of Data Analytics in Product Lifecycle Management
Informed decision-making is crucial for navigating the complexities of product lifecycle management (PLM). Data analytics empowers companies with actionable insights across every lifecycle stage, from design and production to market launch and support. By analyzing performance data, customer feedback, and market trends, businesses can make proactive, data-driven decisions that improve efficiency, reduce costs, and optimize product performance. For organizations looking to stay competitive, data analytics provides a powerful advantage in PLM.
How Data Analytics Enhances Product Lifecycle Decisions
- Improved Demand Forecasting Data analytics allows companies to forecast demand by analyzing sales trends, seasonal patterns, and customer behavior. By accurately predicting demand, businesses can optimize production schedules, reduce inventory holding costs, and minimize the risk of stockouts or overproduction.
- Enhanced Product Quality and Performance Analytics tools track quality metrics and performance data throughout the product lifecycle. By identifying patterns and root causes of defects, teams can make informed decisions to improve quality control processes, minimize rework, and enhance product reliability, leading to greater customer satisfaction.
- Proactive Maintenance and Asset Management Data analytics enables predictive maintenance by monitoring real-time performance and identifying early warning signs of potential failures. Proactive maintenance scheduling reduces unplanned downtime, extends asset life, and lowers repair costs, ensuring that assets are operational and available when needed.
- Optimized Product Development and Design By analyzing customer feedback, market trends, and competitor data, companies gain valuable insights into design and feature preferences. Data-driven design decisions help teams create products that align with customer needs, improving product relevance and reducing the time-to-market for new innovations.
- Enhanced Post-Launch Support and Product Updates Post-launch analytics, such as customer feedback and usage data, provide insights into areas for improvement. Companies can make timely product updates, resolve common issues, and introduce enhancements that increase customer satisfaction and encourage repeat business.
Stages of Product Lifecycle Enhanced by Data Analytics
- Concept and Design Stage In the initial stages, data analytics enables teams to evaluate customer preferences, competitor products, and market trends. This information guides product design, helping companies develop features and specifications that meet customer needs, differentiate from competitors, and minimize redesign efforts.
- Production and Quality Assurance During production, analytics tools monitor metrics such as yield rates, defect counts, and quality inspection results. By analyzing this data in real-time, companies can detect issues early, make process adjustments, and maintain consistent product quality, reducing production costs and waste.
- Sales and Demand Planning Data analytics helps companies forecast sales by analyzing historical data and market conditions. With accurate demand forecasting, businesses can optimize inventory levels, adjust production schedules, and plan effective marketing strategies that align with consumer demand.
- Customer Support and Feedback Analysis After product launch, analytics track customer satisfaction, feedback trends, and usage patterns. This post-launch data enables companies to address customer pain points, implement feature updates, and refine future product development based on real-world insights.
Use Case Examples
- Retail: Optimizing Inventory and Demand Forecasting A retail company used data analytics to forecast demand for seasonal products. By analyzing sales trends and customer purchase behavior, the company adjusted inventory levels to align with expected demand, reducing stockouts and overstock by 30%. This optimized inventory planning improved sales performance and minimized holding costs.
- Automotive: Enhancing Quality Control with Real-Time Data An automotive manufacturer implemented data analytics to monitor defect rates and quality checks across production lines. By identifying defect patterns early, the company adjusted production processes, reducing the defect rate by 20% and improving overall product quality.
- Consumer Electronics: Tailoring Product Updates Based on Customer Feedback A consumer electronics brand used post-launch analytics to track customer feedback and product performance. By analyzing usage data and common issues, the company made targeted updates, improving product features based on customer needs. This data-driven approach led to a 25% increase in customer satisfaction and reduced return rates.
Analytics: Measuring the Impact of Data Analytics on Product Lifecycle Management
- Improvement in Product Quality Companies leveraging data analytics report a 20-30% improvement in product quality, as insights enable them to optimize quality control and address issues early in the production process.
- Reduction in Inventory Holding Costs With accurate demand forecasting and optimized inventory management, businesses experience a 15-20% reduction in holding costs, as products are available in the right quantities at the right time.
- Increase in Customer Satisfaction Data-driven post-launch support and updates lead to a 15-25% increase in customer satisfaction, as companies address customer concerns and introduce enhancements that align with user needs.
Conclusion: Data Analytics as a Key Driver of Lifecycle Optimization
Data analytics provides a strategic advantage in product lifecycle management, enabling companies to make informed decisions, anticipate customer needs, and optimize processes across every stage. From initial design to post-launch support, data-driven insights improve product quality, streamline operations, and increase customer satisfaction. For companies seeking efficiency and competitive differentiation, data analytics is a powerful tool for smarter, faster lifecycle decisions.